Using Big Data and Machine Learning to Predict Poverty and Malnutrition for Targeting, Mapping, Monitoring, and Early Warning
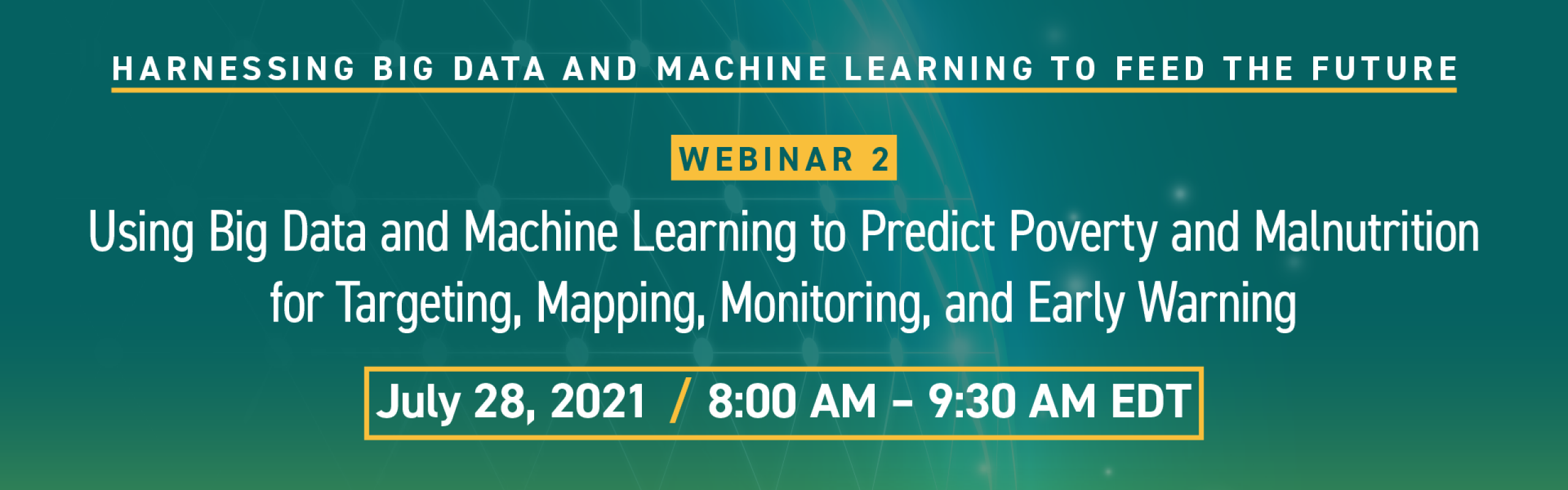
Increasingly plentiful data and powerful predictive algorithms have heightened the promise of data science for humanitarian and development programming. As agencies increasingly embrace and invest in machine learning methods for poverty and malnutrition targeting, mapping, monitoring, and early warning, it is essential to recognize that different objectives require distinct data and methods. In this webinar, we highlight the differences between poverty and malnutrition targeting and mapping, the differences between structural and stochastic deprivation, and the modeling and data challenges of early warning systems development based on machine learning methods. We also present two studies that apply machine learning methods to predict poverty and malnutrition.
This webinar is the second of a two-part webinar to present new data and findings from ongoing research under the United States Agency for International Development (USAID)-funded project "Harnessing Big Data and Machine Learning to Feed the Future", based at Cornell University. Researchers and analysts from operational agencies are invited to join these events for a presentation and discussion of key principles, data sources, methods, and applications.
Linden McBride, St Mary’s College of Maryland, discussed a number of conceptual issues in this research area. Yanyan Liu, IFPRI, presented a study that uses publicly available, moderate-resolution vegetation index (normalized difference vegetation index, or NDVI) data and convolutional neural networks (CNNs) to produce accurate poverty estimates at the community level in low-income, rural economies. Chris Browne, Cornell University, presented a second study that demonstrates how interpretable multivariate random forest models can produce estimates of a set of (potentially correlated) malnutrition and poverty prevalence measures using free, open access, regularly updated, georeferenced data. Finally, Medha Bulumulla, Cornell University, briefly introduced the USAID project web site where data, papers, presentations, and code are freely available and walk participants through an annotated code that can be adapted and replicated for other applications.
Presenters:
- Linden McBride, Assistant Professor, St Mary’s College of Maryland, US
- Yanyan Liu, Senior Research Fellow, Markets, Trade and Institutions Division, IFPRI, USA
- Chris Browne, PhD Candidate, Center for Applied Mathematics, Cornell University, USA
- Medha Bulumulla, Research Assistant, Cornell University, USA
Moderator:
- Christopher B. Barrett, SB&JG Ashley Professor, Cornell University, USA
Discussant:
- Rob Vos, Director of Markets, Trade and Institutions Division, IFPRI, USA