Using Satellite Imagery for Early Warning of Productivity Constraints
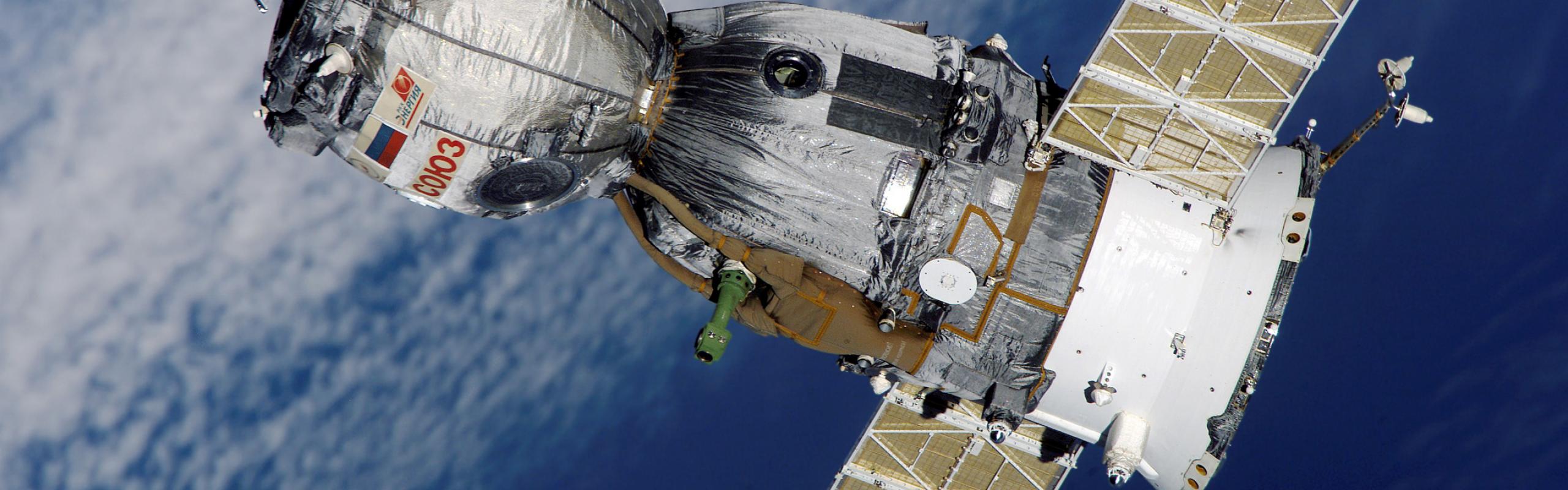
To move the accuracy of food crisis predictions forward, the NASA Harvest Consortium - a multidisciplinary group of applied researchers and operations users led by the University of Maryland - is working to enhance the use of satellite data in decision-making related to food security and agriculture worldwide.
On October 31, 2019, the Consortium in collaboration with the Food Security Portal held a webinar to discuss the potential of satellite imagery for food crisis early warning systems. This webinar provided an opportunity to share the current state of knowledge and to identify areas of new or further collaboration.
Objectives of the Webinar
• Assess the state of the research on predictions of productivity
• Discuss plans for future research
• Host a moderated discussion on the challenges and opportunities that exist
Presentations
Monitoring Yields and Farm Size in Tanzania with Satellite and Drone Data
- Summer Allen, Senior Research Coordinator, IFPRI
- Soonho Kim, Senior Data Manager, IFPRI
Using Satellite Data to Estimate Cropped Area and Yield for African Countries
- Molly Brown, Research Professor, University of Maryland; Science Officer, 6th Grain
Satellite Mapping in Kenya and Nepal for Understanding Yield Variability and Informing Crop Management
- Jake Campolo, Ph.D Student in Earth System Science, Stanford University
Remote Sensing Based Yield Model and Application to Major Exporting Countries
- Belen Franch, Distinguished Researcher, University of Valencia
Intersecting a Global Sub-National Agricultural Statistics Archive with Sub-National Weather, Climate, Food Price, and Population Datasets for Early Warning
- Gary Eilerts, Independent Consultant for NASA
Predicting High-Magnitude, Low-Frequency Crop Losses Using Machine Learning: An Application to Cereal Crops in Ethiopia
- James Warner, Research Coordinator, IFPRI
Discussion moderated by:
- Greg Husak, Assistant Researcher, University of California, Santa Barbara (UCSB)
We acknowledge support to this webinar from the NASA Harvest Consortium (NASA Applied Sciences Grant No. 80NSSC17K0625) .
If you have any additional questions and/or comments about the webinar or for the presenters specifically, please send an email to ifpri-fsp@cgiar.org.